How is technology changing the way robots ‘see’ and perceive the world? Michelle Mooney asks the experts.
Robotic perception is at the forefront of transformative change, redefining how machines interact with their surroundings and process complex data. In an era where automation extends beyond industrial confines into diverse fields such as education, underwater exploration, and logistics, the ability of robots to perceive and understand their environment has become a fundamental determinant of their success. Here, Robotics & Automation Magazine brings together insights from industry leaders who are navigating the intricate challenges and breakthroughs associated with developing advanced perception systems.
Louis Esquerre-Pourtere, head of research and development at Exotec, discusses the essential role of designing hardware and algorithms that adapt to fluctuating conditions, ensuring seamless, 24/7 operations in demanding environments; Dr Farshad Badie, dean of faculty of computer science and informatics at the Berlin School of Business and Innovation, sheds light on integrating multi-sensor data fusion for interactive, real-time applications in educational settings; and Coena Das, robotics engineer at the National Robotarium, highlights the unique challenges faced in underwater robotics, where traditional sensors fall short, requiring novel solutions such as acoustic imaging.
Together, they explore a range of sensor technologies, the balance between processing speed and accuracy, and the integration of AI and machine learning, to understand the strides and hurdles in crafting robots capable of perceiving their environments as dynamically as humans do.
What are the key challenges your company faces in developing robot perception systems?
Louis Esquerre-Pourtere: Perception is the first step in robot movement; it is essential that a robot should have a clear understanding of its environment, this allows it to make the right decision, especially if it is autonomous. At Exotec we see several key elements that can be challenging regarding perception. One of these is the environment the robot operates in. The solution, in this case, the robot, must be reliable and the service provider must consider the possible variations of the environment such as light, temperature, dust, floor levelling, and many more environmental considerations. All those parameters need to be taken in account in both the hardware design and the algorithm of the robot to provide an end- to end solution that will be compatible with a maintenance-free 24/7 usage. Other aspects to consider are precision and performance: the perception solution must be designed according to the robot position needs to find the right balance between precision and computing time. Bad choice in the hardware design can lead to non-competitive solution or to a solution that is unreliable and causes problems onsite.
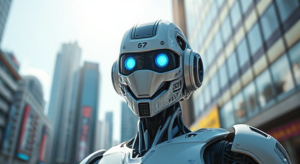
Dr Farshad Badie: One of the key challenges we face when developing robotic perception systems is creating robust systems that can seamlessly adapt to dynamic environments, such as educational settings where student interactions vary. Ensuring that our robot, BOTSBI can accurately interpret and respond to complex student inquiries in real-time is another challenge, as it requires sophisticated natural language processing and a deep understanding of context. Additionally, integrating BOTSBI with our diverse virtual learning environments (that operate across multiple platforms) presents technical hurdles in terms of compatibility and real-time responsiveness. Getting perception wrong here can lead to all kinds of problems with general intelligibility.
Coena Das: Sensor limitations are a big problem. Even the most advanced sensors have inherent limitations that must be addressed. These include range constraints that limit the robot’s perception distance, resolution issues that affect the detail of sensed data, and interference problems that can arise from environmental factors or other nearby sensors. Another common issue is that of real-time processing. One of the most critical challenges is achieving a balance between the accuracy of perception and the computational resources required for real-time processing. High-accuracy algorithms often demand significant processing power, which can lead to delays in decision-making. Furthermore, there are many data challenges that make the widespread implementation of robotic perception systems difficult right now, which is why they can only be used in limited circumstances. The development of robust perception systems relies heavily on diverse and high-quality training datasets. Obtaining such datasets can be time-consuming and expensive, particularly for specialised or rare scenarios. Additionally, the process of annotating this data accurately is labour-intensive and prone to human error. This means we still have a long way to go in perfecting this process.
BOT BYTE: The global market for machine vision systems is projected to reach £12bn by 2025, reflecting a CAGR of 7.6% from 2020. Source: Fortune Business Insights
Which sensor technologies do you rely on most for robot perception, and how do you decide which to use for a specific application?
LEP: When we are coming up with a strategy to decide which technology will be used, we consider a variety of options. Lidar is a well-established robotic perception technology that has been researched for years. It is highly predictable and reliable, but it is limited to 2D mapping and its precision depends on the number of points and the refresh rate of the data. This is very useful in environments where topology is known or to detect ‘object’ without the need for precise object definition. Edge cases such as extreme reflexion on corners, specific materials or finish must be considered. Another technology we consider when looking for the right solution is radar. This is a very efficient technology but can be seen as a ‘one-point lidar’. Radar perception technologies are very compact, cost effective and perturbations are limited, but the technology requires more integration to gather a complete mapping of the scene for the selected robot. Next, we think about cameras. Whether mono or stereo, together with image processing, these allow us to manage many applications, but they require a lot of training and processing resources for mobile robots especially when high resolution images are used and needed for robot precision. Ultimately there is not a magic technology for applications, and we like to compile different data sources to reach what is expected of us from a client.
How does your company handle the fusion of data from multiple sensors to enhance the robot’s understanding of its environment?
FB: To enhance BOTSBI’s interpretation as well as comprehension of its environment, the best strategy would be to employ a multi-sensor fusion approach. This involves integrating data from various sources, such as audio, visual and motion sensors that allow the robot to ‘interpret’ its surroundings more accurately. By combining these inputs, we aim at improving BOTSBI’s ability to respond intelligently to both verbal (i.e., linguistic) and physical cues, creating a more interactive and engaging educational experience. This fusion can be managed through advanced algorithms that prioritise real-time processing and contextual accuracy, ensuring a smooth and intuitive user experience.
Are there specific environments or tasks that are more difficult to perceive accurately?
CD: Our underwater robotics work comes with many challenges that test the limits of regular perception methods. Visibility in underwater settings is often severely compromised due to murky water conditions or the absence of natural light at greater depths. This necessitates the deployment of advanced sonar systems or sophisticated acoustic imaging technologies to navigate and perceive the environment effectively. These specialised tools allow our robots to ‘see’ in conditions where traditional optical systems would be rendered ineffective.
Beyond visibility issues, underwater environments pose additional challenges that impact the entire robotic system. Corrosion becomes a significant concern, as prolonged exposure to saltwater can degrade sensors and electronic components. To combat this, we implement robust protective measures and utilise corrosion resistant materials in our designs. The immense pressure at greater depths also necessitates the development of pressure-resistant housings for all electronic components, including cameras and other sensitive equipment.
BOT BYTE: The agricultural sector has seen a 25% increase in the adoption of robots with advanced perception systems. Source: Robotics and AI for Precision Agriculture
Waterproofing is another critical aspect of our underwater robotics work. Every electronic component, connection, and enclosure must be meticulously sealed to prevent water ingress, which could lead to catastrophic system failures. This adds layers of complexity to the design and maintenance of our underwater robots. The challenge of recreating underwater environments for testing and development purposes is also substantial. Simulating the unique conditions of underwater settings, including pressure, visibility and water movement, requires specialised facilities and innovative approaches to ensure that our perception systems are adequately prepared for real-world deployment.
How important is real-time processing in your robot perception systems? And how do you balance the need for speed versus the accuracy of perception data?
LEP: Real-time decisions are critical for mobile robot applications. When a robot is moving at four metres per second, a few milliseconds can make the difference between a robot stop and a robot crash. Precision and time processing are two inputs for two different usages. One is accuracy and is defined according to robot position constraints and should be limited to what is needed in order not to impact the processing time. Processing is additionally defined according to the action chain – in other words, according to the performance you need to reach. You need to balance costs or choose to impact the performance of your product.
FB: Real-time processing is critical in BOTSBI’s perception system – especially in educational contexts where immediate responses are needed to keep students engaged. For instance, when delivering scientific presentations or, in the future, answering students queries one by one, delays in processing disrupt the flow of interaction and diminish the learning experience. Therefore, BOTSBI’s design has been designed in a way to prioritise efficient real-time processing to ensure smooth, responsive, and meaningful engagement with users.
What role does machine learning (ML) or artificial intelligence (AI) play in improving your robot’s perception abilities? Do you rely on pre-trained models, or do you develop custom models in house?
LEP: ML is very effective and useful when facing situations when the environment cannot be controlled. In the warehouse, typical ML/AI usage is used for automatic picking. The first step of identifying what to pick is complex; it requires the software to identify the correct object and which container it needs to go into, then it must select the best option to pick. As there is a lot of variation in term of object, number, location, material, ML and AI are great technologies to use to determine the best choice. Once the software does its job, the robotic arm can do its job.
FB: ML and artificial AI play the most fundamental roles in continuously improving BOTSBI’s perception capabilities. Through AI, BOTSBI learns from interactions with students and faculty, refining its natural language processing to better understand complex questions and contextual nuances. ML models allow BOTSBI to improve its visual recognition and predictive capabilities, enabling it to adapt to new environments, anticipate student needs, and provide more accurate and personalised responses over time.
CD: In a recent project focused on visual inspection, we leveraged both conventional and ML-based approaches for our perception pipeline. Initially, we used traditional computer vision techniques to detect specific features like edges and corners, which worked well for simple alignment tasks. However, as we encountered more complex and dynamic attributes to the tasks, we found these methods struggled to scale effectively. To address this challenge, we implemented modern machine learning models, particularly convolutional neural networks, for automatic feature identification and extraction. These models, pre-trained on large datasets of industrial components and assemblies, proved to be much more adaptable. They required less manual tuning of specific target features and demonstrated impressive generalisation across various types of alignment inspection tasks.
This shift to ML-based perception significantly improved our system’s ability to handle diverse components and varying lighting conditions on our artefacts. It also reduced the need for extensive reprogramming when introducing new products or slight variations in existing ones, greatly enhancing the flexibility and efficiency of our visual inspection process.