MIT researchers have for the first time enabled a soft robotic arm to understand its configuration in 3D space, by leveraging motion and position data from its own ‘sensorised’ skin.
Soft robots constructed from highly compliant materials, similar to those found in living organisms, are being championed as safer, and more adaptable, resilient, and bio-inspired alternatives to conventional rigid robots.
However, MIT said giving autonomous control to these deformable robots is “a monumental task” because they can move in a virtually infinite number of directions at any given moment. That reportedly makes it difficult to train planning and control models that drive automation.
Conventional methods to achieve autonomous control use large systems of multiple motion-capture cameras that provide the robots feedback about 3D movement and positions. However, according to MIT, those are impractical for soft robots in real-world applications.
In a paper being published in the journal IEEE Robotics and Automation Letters, the researchers describe a system of soft sensors that cover a robot’s body to provide “proprioception” — meaning awareness of motion and position of its body.
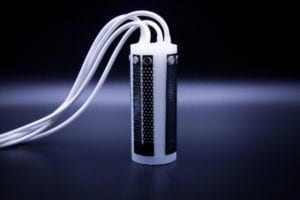
That feedback runs into a novel deep-learning model that sifts through noise and captures clear signals to estimate the robot’s 3D configuration.
The researchers validated their system on a soft robotic arm resembling an elephant trunk, that can predict its own position as it autonomously swings around and extends.
Ryan Truby, a postdoc in the MIT Computer Science and Artificial Laboratory (CSAIL), said: “We’re sensorising soft robots to get feedback for control from sensors, not vision systems, using a very easy, rapid method for fabrication.
“We want to use these soft robotic trunks, for instance, to orient and control themselves automatically, to pick things up and interact with the world.
“This is a first step toward that type of more sophisticated automated control.”
According to Daniela Rus, director of CSAIL, one future aim is to help make artificial limbs that can more dexterously handle and manipulate objects in the environment.
“Think of your own body: You can close your eyes and reconstruct the world based on feedback from your skin,” says Rus.
“We want to design those same capabilities for soft robots.”
Next, the researchers aim to explore new sensor designs for improved sensitivity and to develop new models and deep-learning methods to reduce the required training for every new soft robot.
Currently, the neural network and sensor skin are not sensitive enough to capture subtle motions or dynamic movements, however they hope to refine the system to better capture the robot’s full dynamic motions or learning-based approaches to soft robotic control.